ECDA Insights Social Artificial Intelligence #6

- Dit evenement is voorbij.
ECDA Insights Social Artificial Intelligence #6
16 juni 2022 @ 16:00 – 17:00
Presenter: Max Welz
Title: “Identifying Periods of Careless Responding in Surveys: A Deep Learning Approach”
Abstract: Rating-scale datasets collected from surveys are paramount to empirical research. However, due to deficiencies in survey design or lack of motivation, some respondents may not comply with the instructions of the survey’s questions. This phenomenon is known as careless responding. Careless responding has been identified as a major threat to the internal validity of survey-based studies and should therefore be screened for (Huang et al., 2015). Existing methods for detecting careless responses are designed to identify respondents who respond carelessly throughout the survey. However, recent work suggests that the longer a survey takes, the higher the likelihood that a large proportion of all respondents will eventually start responding carelessly (Bowling et al., 2021). Thus, we are interested in identifying when a respondent becomes careless (if at all) rather than trying to detect respondents who respond carelessly throughout the survey. Correspondingly, we propose a novel method for identifying the periods of carelessness (or a lack thereof) of each respondent. The proposed method uses the deep learning technique of auto-associative neural networks (autoencoders) in combination with response times. By means of extensive numerical experiments, we find that our proposed method achieves high reliability in correctly identifying periods of careless responding and discriminates well between careless and regular respondents. Our method seems to perform particularly well in long surveys, which are common in psychology and health sciences, where it is likely that a large proportion of all respondents eventually respond carelessly due to fatigue.
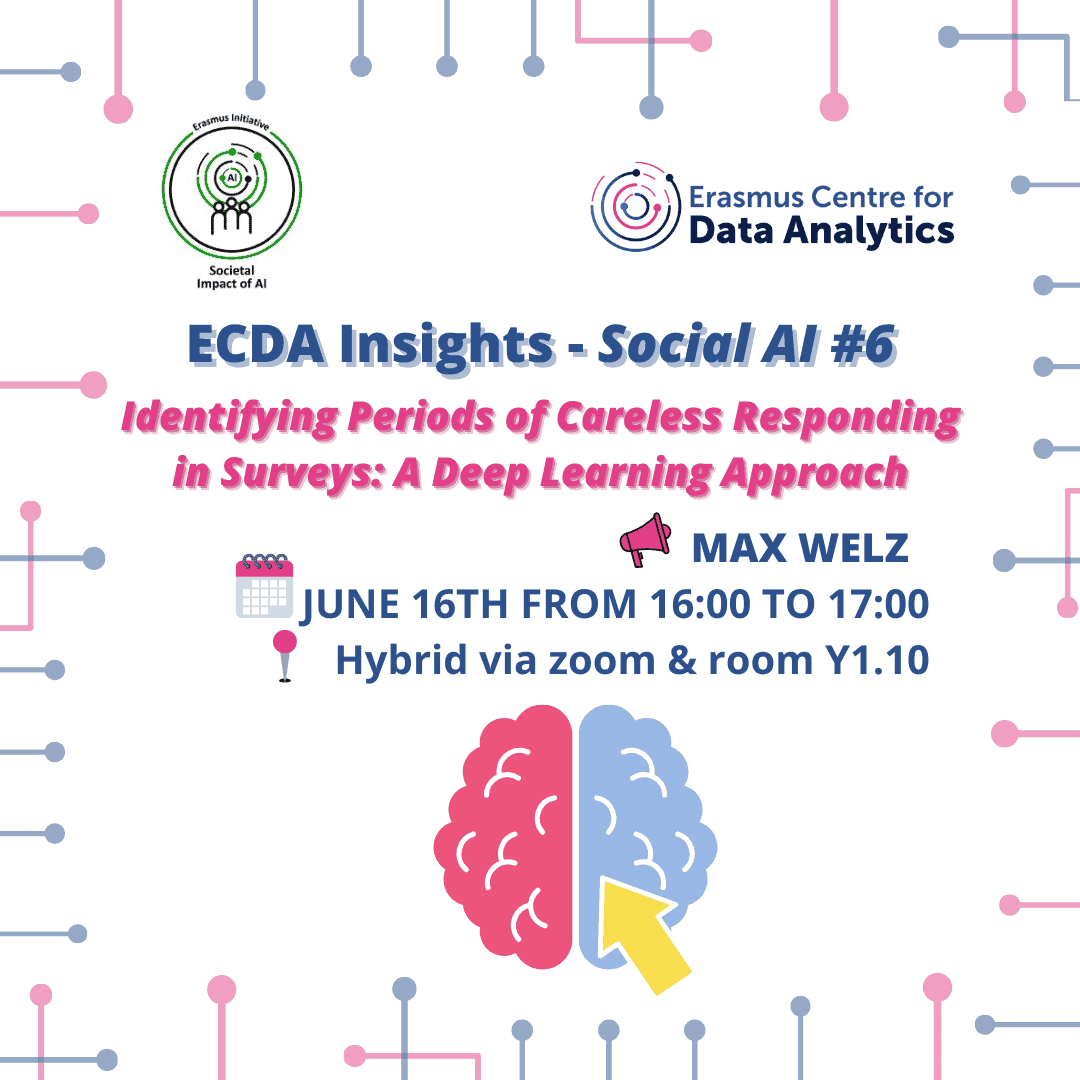